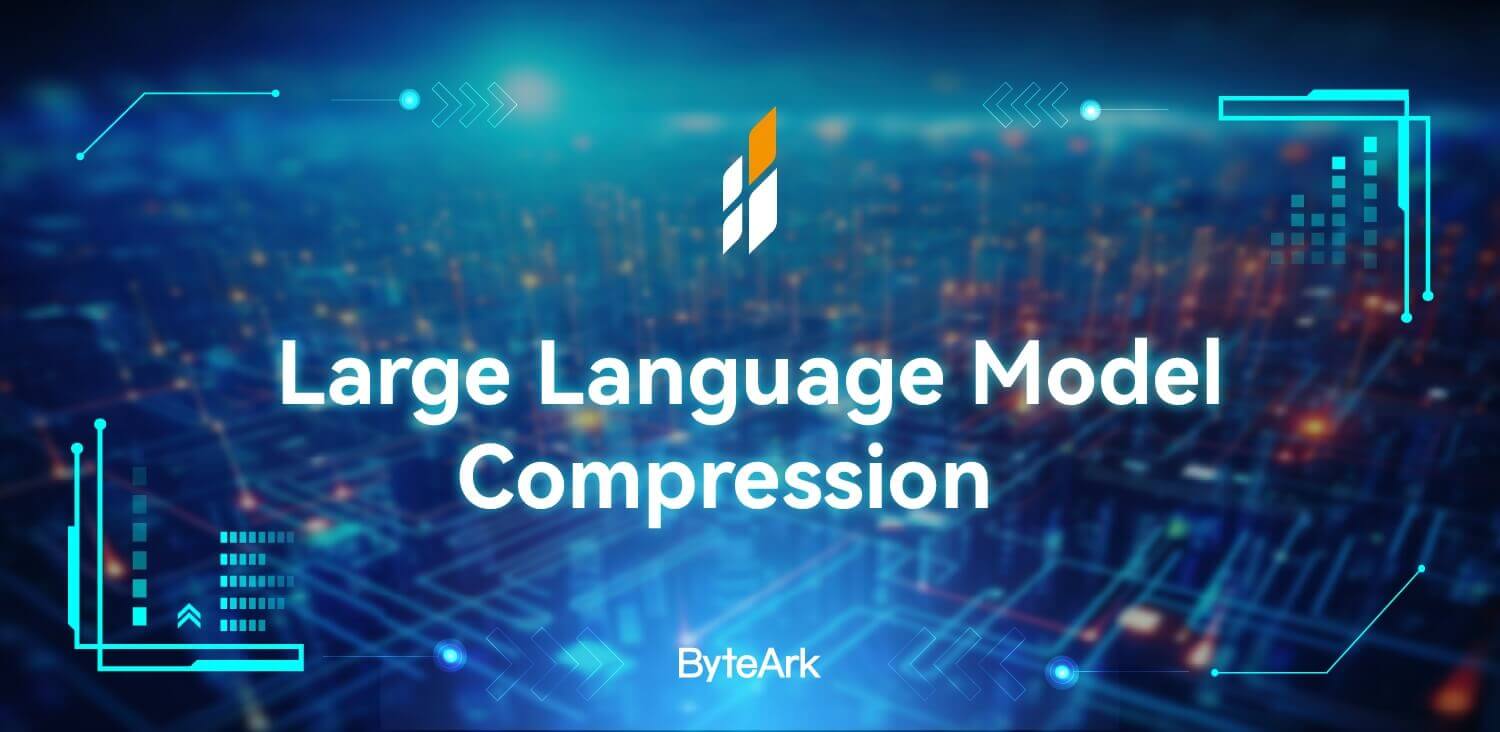
In this work, we tackle the critical challenge of compressing large language models (LLMs) to facilitate their practical deployment and broader adoption. We introduce a novel post-training compression paradigm that focuses on the low-rank decomposition of LLM weights. Our analysis identifies two main challenges in this task: the variability in LLM activation distributions and handling unseen activations from different datasets and models.
To address these challenges, we propose a nested activation-aware framework (NSVD) for LLMs, a training-free approach designed to enhance the accuracy of low-rank decompositions by managing activation outliers by transforming the weight matrix based on activation distribution and the original weight matrix.
This method allows for the absorption of outliers into the transformed weight matrix, improving decomposition accuracy. Our comprehensive evaluation across eight datasets and six models from three distinct LLM families demonstrates the superiority of NSVD over current state-of-the-art methods, especially at medium to large compression ratios or in multilingual and multitask settings.
First, we evaluate the performance of LLaMA-7B compressed using NSVD (here, $k_1=0.95k$) and baselines under compression ratios ranging from 10% to 50% across all eight datasets.
Our results include comparisons with ASVD-II, NSVD-I, and NSVD-II; since no improvements were observed using the proposed ASVD-III method, its results are omitted for brevity. Table 1 summarizes these findings. We observe that ASVD-I and ASVD-II yield equivalent performance when ignoring numerical errors. Similarly, NSVD-I and NSVD-II also produce comparable outcomes.
NSVD-I or NSVD-II consistently outperforms standard SVD, ASVD-0, and ASVD-I across all the compression ratios.
More importantly, NSVD exhibits significant advantages over baselines under medium to high compression ratios. Specifically, at a 30% compression ratio, compared to the best-performing baseline, NSVD-I reduces perplexity on PTB, C4, SNIPS, AlpacaEval, MCTest, CMRC (CN), and AlpacaEval (JP) by 7.1%, 5.4%, 12.1%, 6.3%, 1.3%, 16.1%, and 54.8%, respectively; when the compression ratio reaches 40%, NSVD can reduce perplexity by more than 60%.