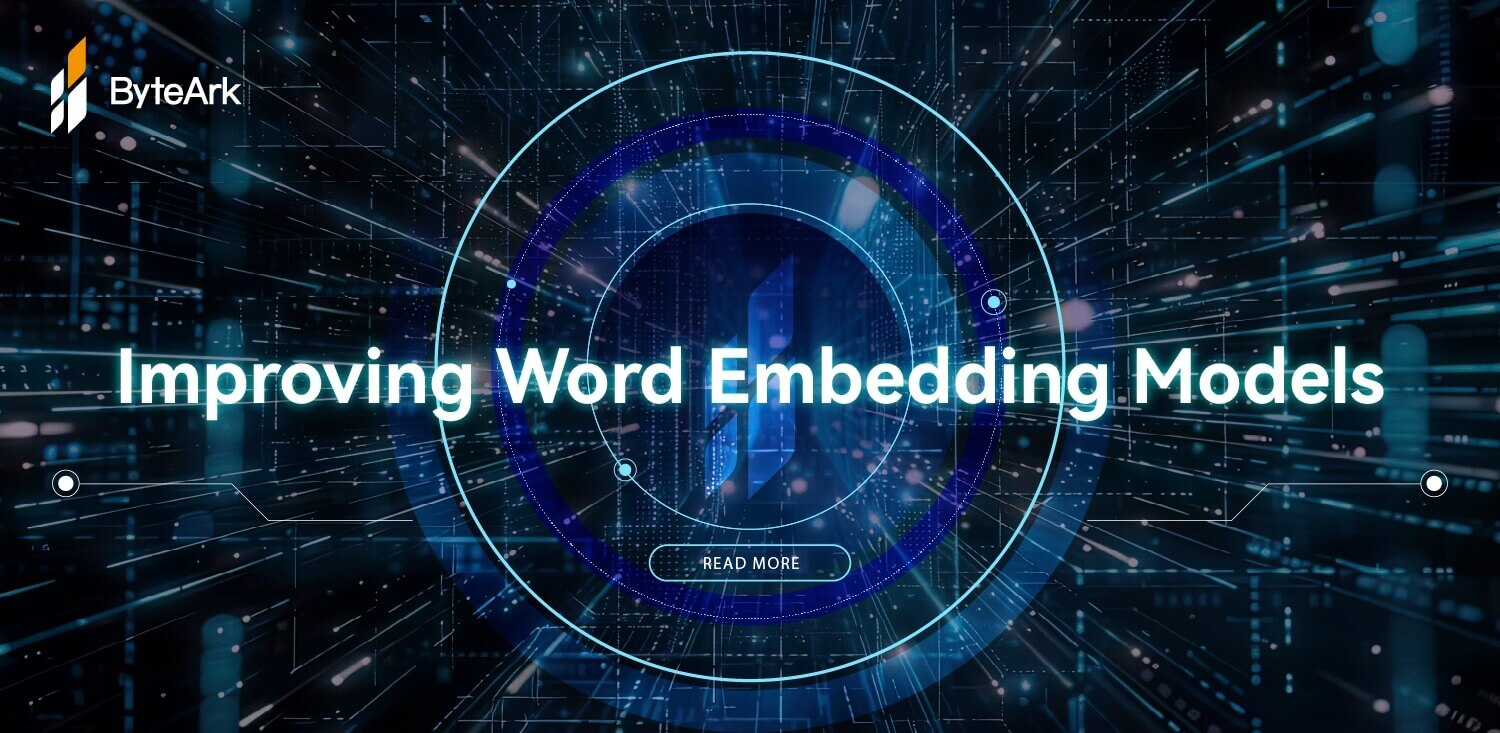
This work presents an approach to improve text embedding models through contrastive fine-tuning on small datasets augmented with expert scores. It focuses on enhancing semantic textual similarity tasks and addressing text retrieval problems. The proposed method uses soft labels derived from expert-augmented scores to fine-tune embedding models, preserving their versatility and ensuring retrieval capability is improved. The paper evaluates the method using a Q&A dataset from an online shopping website and eight expert models. Results show improved performance over a benchmark model across multiple metrics on various retrieval tasks from the massive text embedding benchmark (MTEB). The method is cost-effective and practical for real-world applications, especially when labeled data is scarce.
Table 1 and 2 present the evaluation of nDCG@10 and mAP@10 metrics, respectively, for different models across various datasets from MTEB retrieval tasks. The average nDCG@10 scores for Benchmark, Soft-1, Soft-2, and Hard label models are 39.675, 40.633, 40.334, and 37.574, respectively, with standard deviations of 29.963, 28.552, 28.167, and 27.081, respectively. And the average mAP@10 for Benchmark, Soft-1, Soft-2, and Hard label models are 34.419, 35.323, 35.04, and 32.243, respectively, with standard deviations of 29.693, 28.587, 28.221, and 26.585, respectively. The win rate of Soft-1 over the Benchmark is 50.37% in terms of nDCG@10, and is 55.38% with respect to mAP@10. This again confirms that no single text embedding method dominates across all tasks (Muennighoff et al., 2022). The Soft-1 and Soft-2 models demonstrate promising results with higher scores and smaller standard deviations compared to the Benchmark model, suggesting they perform well across various datasets and their performance is consistently stable. The Hard-label model, on the other hand, has worse nDCG@10 and mAP@10 scores compared to the Benchmark; although it has a smaller standard deviation. The improvement seen in the fine-tuning with Soft-1 and Soft-2 labels might be attributed to the reduced anisotropy in the fine-tuned models (meaning the text embeddings occupy a larger cone in the vector space after fine-tuning). This property is further supported by the results on the held-out set: the Soft-1 and Soft-2 models have better results in terms of area under precision-recall (PR) curve (see Section 4.3). The text embeddings of irrelevant pairs are then distributed across a wider range of the vector space.